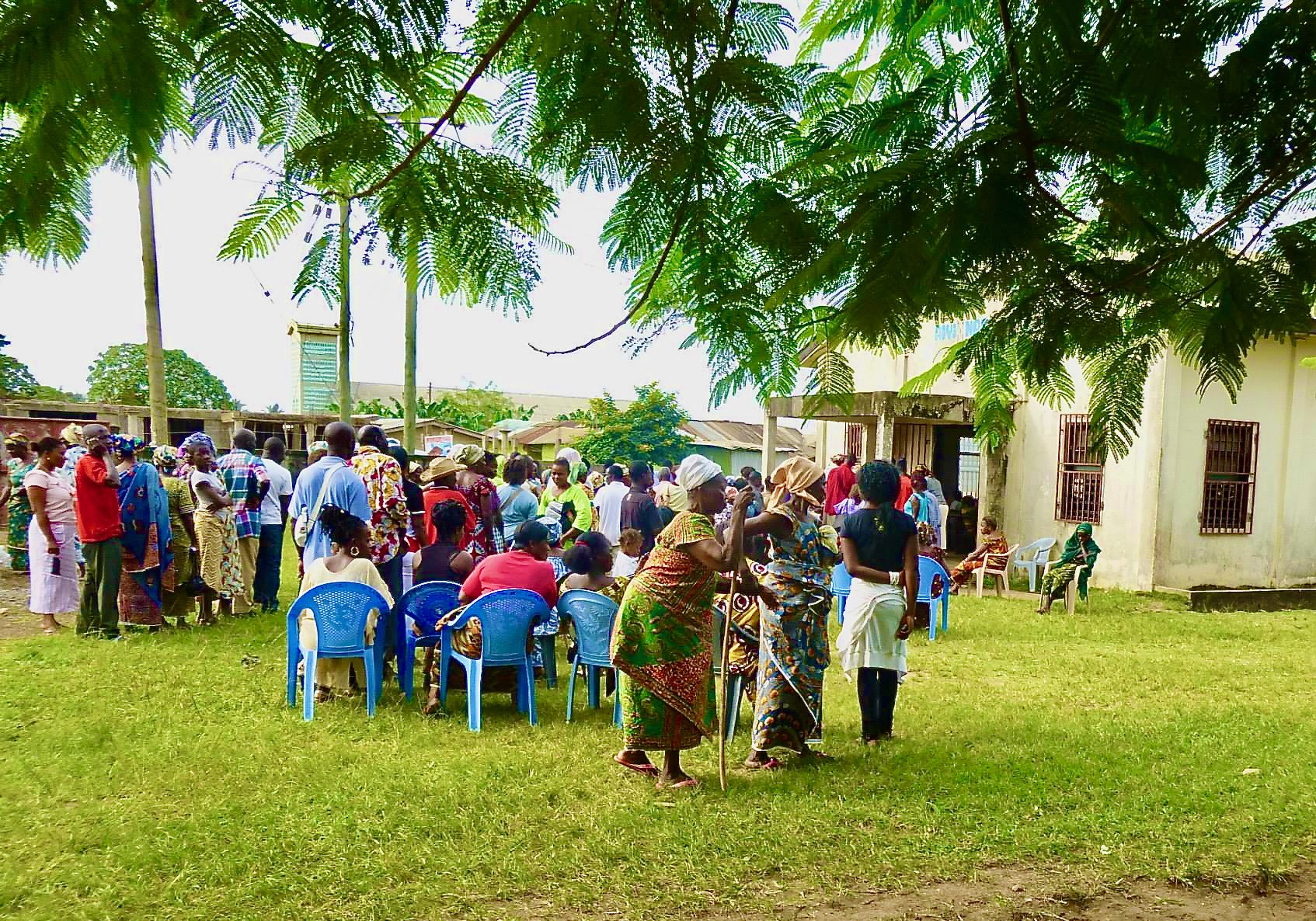
MONITORING AND EVALUATION CERTIFICATE
Module 12: Evaluation Study Designs
Qualitative Design
It is uncommon for evaluators to use only qualitative methods when evaluating a program. Some clients are more at ease with making decisions based on quantitative data outputs, while some understand the significant costs and time associated with qualitative data collection. It is also very difficult to generalize to other populations based on qualitative data, which therefore makes program replication and scale-up more difficult. On the other hand, supporters of qualitative evaluations believe that context is such a large factor in program implementation success that generalizing to other populations is not possible despite the methodology choices.(1) However, qualitative evaluation methods are extremely beneficial in providing rich program feedback and evaluators should consider integrating them into the evaluation plan by using a "mixed methods" approach. For more information on qualitative data collection methods to incorporate into program evaluations, please refer to the certificate in Global Health Research.
Quantitative Designs
If clients or stakeholders are interested in a quantitative evaluation plan, there are different design sequences that can be used, depending on time, money, and availability of data. Linking directly to the needs of the stakeholders and the purpose of the evaluation, study designs can either be experimental (with a traditional control group), quasi-experimental (with a comparison group that may not necessarily be a control group), or non-experimental (where no formal control or comparison group exists).(2)It is important to note how evaluation differs from monitoring; monitoring data like monthly reporting forms, stock outs for supply chain management, and some disease tracking is done consistently and frequently throughout the life of a project. Please refer to http://www.uniteforsight.org/metrics-course/monitoring-evaluation for more information on the differences between monitoring and evaluation processes.
In many cases, it is impossible to randomly assign individuals into experimental and control groups, as is often done in clinical or randomized control trials (RCT). When RCT is unavailable, comparison groups are selected that match the target population on a number of population characteristics, closely resembling the group that receives the intervention. This is the main difference between experimental and quasi-experimental designs.(3) For example, if the intervention is held in a school, a classroom with students of similar academic level that is not receiving the intervention may be used as a comparison group; this ensures that the children in the intervention and comparison groups have had somewhat similar school experiences, are most likely the same age range, and may live in the same geographic area.(4)
Quasi-experimental design (QED) is the most common quantitative design in global health evaluation. There are various schedules of data collection, differing in level of robustness according to time, money, and availability of data (see: Constraints on Evaluation).
It is recommended that QED data collection occur at four different time points: pre-intervention, mid-intervention, post-intervention, and ex-post intervention. Pre-intervention data (also referred to as baseline data) is collected on relevant evaluation indicators prior to the intervention. Evaluators and program implementers look for changes in the intervention group from pre- to post-intervention data, signaling the possibility of project impact. Baseline data may also be collected far in advance of program development as a way to determine the needs of the community in more participatory approaches. Mid-intervention measures occur around the mid-point of intervention, which will vary according to the timeline of intervention. Post-intervention data is collected immediately after the intervention has ended, and ex-post data is collected much later, possibly 5 to 10 years after the intervention has ended, in order to assess the impact or sustained effects of the program.(5) At each of these times, data may be collected from the intervention group, the comparison group, or both. The source and time of data collection will depend on the level of available resources and the needs of the evaluation.
In the most ideal situation with surplus resources, evaluators would collect data with consistent methods on relevant indicators from both groups at all four times. Without any randomization as in experimental designs, this design is the closest to a randomized control trial and can be visually represented as follows:
The "X"s represent data collected at that time point.
Various time, budget, and data constraints often prevent the use of this robust and extensive schedule of health program evaluations. First, evaluators may not be introduced into the process until the intervention is already in place, eliminating the opportunity to collect baseline data unless there was a pre-existing record. Second, budgets may not allow for mid-intervention measurement nor guarantee ex-post measurement, which may be considered less critical than baseline and post-intervention measures for determining project outcomes. Third, depending on how large the required sample size is, the collection of data that may be considered non-essential may be removed for the sake of balancing a budget with a significant sample size. Also, budget, time, and data constraints may eliminate the possibility of a comparison group: a valid comparison group may not exist, there may not be sufficient funding for comprehensive data collection, or late intervention may preclude the creation of a comparison group. As the number and type of measurements are reduced, the model of data collection becomes less robust and more susceptible to invalidity.(6)
The least robust QED is represented by just a post-intervention measure in the intervention group, shown below:
Occurring frequently in global health evaluation, this schedule does not show demonstrative change in the intervention group nor impact of intervention when evaluated against a comparison group —two main goals of evaluation.(7) Instead, evaluators collect data on participants in the program (those who have received the intervention) and attempt to make the most relevant and conclusive statements about the success of the program. At this stage, evaluators may choose to supplement quantitative data with qualitative methods in order to bolster the findings. Recall is one strategy for estimating a rough baseline (e.g. asking people what their income used to be or how many times they went to the doctor five years ago), however, it creates a risk of bias and inaccuracy if not triangulated with other forms of data collection methods. This is an appropriate place to incorporate qualitative methods to triangulate and reinforce the recalled data, quantitatively, in the post-intervention period.(8) With only one measurement and no effective comparison group, this particular study design may also be categorized as non-experimental.
Hypothetical Case: Evaluating Demand for a Health Program(9)
If an evaluator is only interested in looking at the demand for a specific health program (for example, safe-sex education), as well as what kind of people are attending the related program lectures, they may perform a post-test only design among the intervention group. After conducting a number of health education sessions for a population, the evaluator may survey the attendants and ask various questions to determine why they chose to come to the sessions, along with standard demographic information collection. In this situation, the data collected would most likely be formative in the sense that the evaluator would report this information to program developers and they could determine the best health education program for this population based on the evaluation reports.
In between the most and least robust study designs, there are other options that may suit available resources; evaluators and clients may discuss which measurements they believe are most crucial to determining program success (or obtaining continued funding) and proceed from there. It should be noted, however, that if limited resources exist, it is typically better to do one measurement each in a comparison and intervention group than take measurements at two different times from only the intervention group. For example, it is (usually) better to do this:
than this:
The latter works well when the theory in practice is well established or the client and evaluators are only interested in determining adequacy of the intervention. However, in pilot programs or evaluations that seek to determine probability or plausibility, only measuring twice among the intervention group is not sufficient. As discussed in the Purpose of Evaluation module, probability and plausibility evaluations are more involved but have the ability to derive more pertinent and significant program information than adequacy assessments. By collecting indicator data from both the comparison and intervention groups, differences can better estimate how well the project can be generalized to other populations.(10) However, in both cases, the final decision for the study design will come down to the ability to access a comparison group and the aim of the evaluation.
Threats to Validity
Evaluations become less robust and less valid as the number of measurements is reduced and the comparison group is compromised. In particular, the amount of control in an evaluation determines the level of internal validity.(11) Consequently, the evaluation schedule with a single post-test measurement of the intervention group would have little internal validity because there are few control measures in place (i.e no comparison group, limited measurements, no randomization).
When only one group is measured, evaluation risks shortcomings due to "maturation." This is the risk that evaluation indicators are measuring only growth that would have occurred naturally through the maturation process, rather than growth due to program activities.(12) For instance, measuring math skills of 5th graders in a tutoring program will most likely produce improved indicators simply because of the normal educational process and brain function, not necessarily the impact of the tutoring program. History threats are similar and reflect events or changes in the surrounding environment that may have altered the results, and so the results cannot be attributed to program activities alone.(13) For example, if a country is improving the economy and spending more on healthcare, it is possible that a concurrent decline in child mortality could have been attributable to these external factors rather than to a specific intervention. It is therefore necessary to examine potential confounding variables and processes of maturation in the final evaluation.
Footnotes
(1) Bamberger, M., Rugh, J., and Mabry, L. (2006). Real world evaluation: Working under budget, time, data, and political constraints. Thousand Oaks, CA: Sage Publications, Inc.
(2) The World Bank. (n.d.). Impact evaluation: Evaluation designs. Poverty Reduction and Equity.
(3) Gribbons, B., and Herman, J. (1997). True and quasi-experimental designs. Practical Assessment, Research and Evaluation, 5(14).
(4) Harrell, A., Burt, M., Hatry, H., Rossman, S., Roth, J., and Sabol., W. (n.d.). Evaluation strategies for human service programs: A guide for policymakers and providers. Washington, DC: The Urban Institute.
(5) United Nations World Food Programme. (n.d.). Monitoring and evaluation guidelines: What is RBM oriented M&E? Rome, Italy: United National World Food Programme Office of Evaluation and Monitoring.
(6) Bamberger, M., Rugh, J., and Mabry, L. (2006). Real world evaluation: Working under budget, time, data, and political constraints. Thousand Oaks, CA: Sage Publications, Inc.
(7) Shadish, W.R., Cook, T.D., and Campbell, D.T. (2002). Experimental and quasi-experimental designs for generalized causal inference. Boston, MA: Houghton Mifflin.
(8) Bamberger, M., Rugh, J., and Mabry, L. (2006). Real world evaluation: Working under budget, time, data, and political constraints. Thousand Oaks, CA: Sage Publications, Inc.
(9) Fisher, A. and Foreit, J. (2002). Designing HIV/AIDS intervention studies: An operations research handbook. Washington, DC: Population Council.
(10) Ibid.
(11) Slack, M.K. and Draugalis, J.R. (2001). Establishing validity of experimental studies: Threats to internal validity. American Journal of Health-System Pharmacy 58(22).
(12) Trochim, W.M.K. and Donnelly, J.P. (2006). Research methods knowledge base. Cengage Learning.
(13) Issel, L.M. (2009). Health program planning and evaluation: A practical, systematic approach for community health (2nd ed.) Sudbury, MA: Jones and Bartlett Publishers.